Authors: Mohammad Sadegh Akhondzadeh, Alireza Omidi, Zeinab Maleki, Kevin Coombes, Amanda E. Toland, Amir Asiaee
Published in ICML Workshop on Computational Biology, 2020
Abstract
We model the partial order of accumulation of mutations during tumorigenesis by linear structural equations. In this framework, the cancer progression network is modeled as a weighted directed acyclic graph (DAG), which minimizes a suitable continuous loss function. The goal is to learn the DAG from cross-sectional mutation allele frequency data. As a case study, we infer the order of mutations in melanoma. The recovered network of melanoma matches the known biological facts about the subtypes and progression of melanoma while discovers mutual exclusivity patterns among mutations by negative edges.
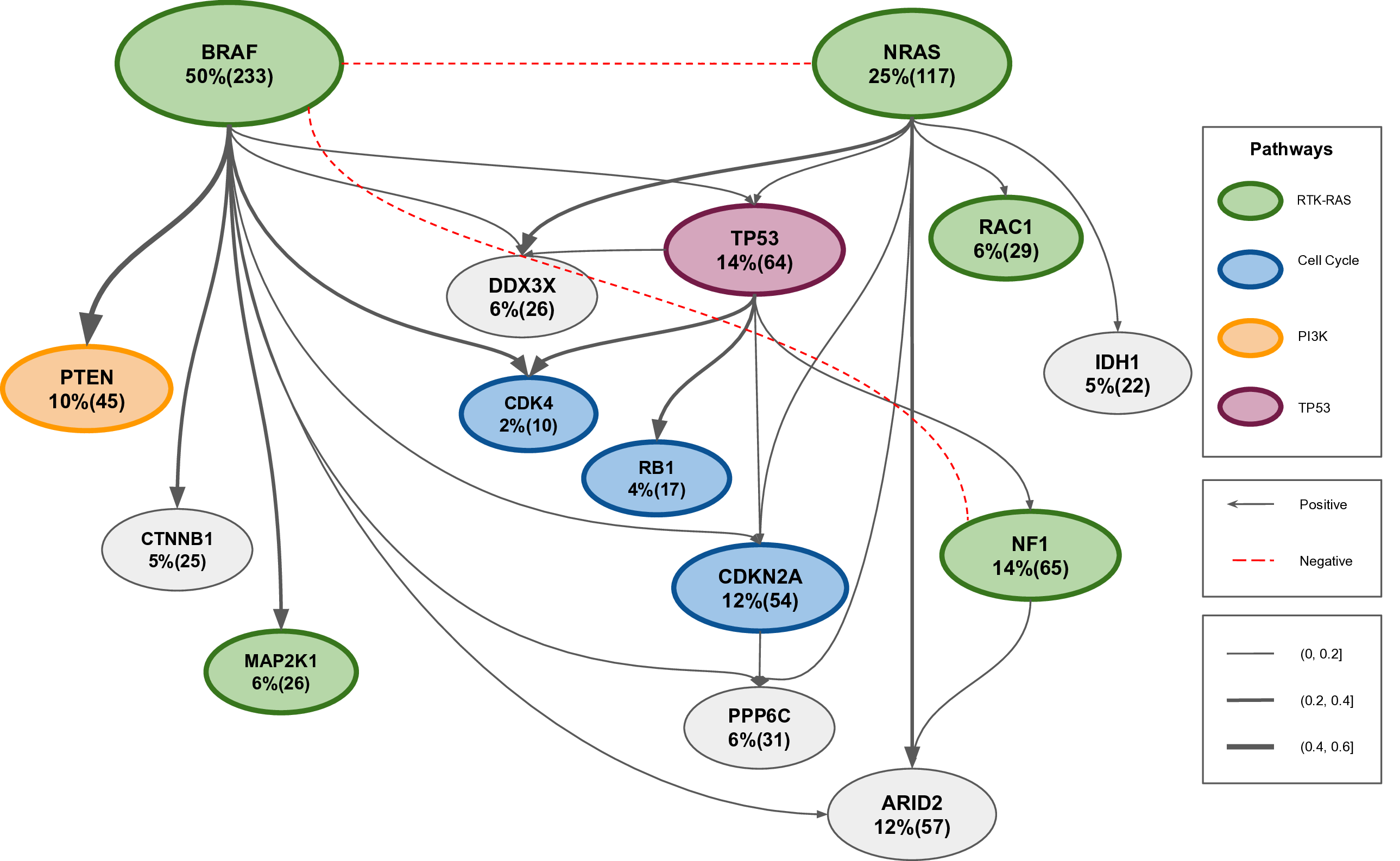
Figure 1. The learned cancer progression network of melanoma